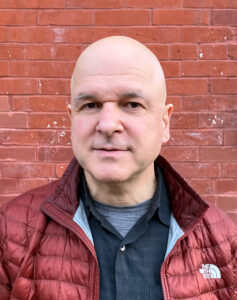
Abstract
Meaning by Courtesy: LLM-Generated Texts and the Illusion of Content
Department of Medical Education, Icahn School of Medicine at Mount Sinai, New York, NY, USA
Department of Philosophy, Graduate Center, CUNY, New York, NY, USA
E-mail: gary.ostertag@mssm.edu
Recently, Mann et al.1 proposed the use of “personalized” Large Language Models (LLMs) to create professional-grade academic writing. Their model, AUTOGEN, is first trained on a standard corpus and then “fine-tuned” by further training on the academic writings of a small cohort of authors. The resulting LLMs outperform the GPT-3 base model, producing text that rivals expert-written text in readability and coherence. With judicious prompting, such LLMs have the capacity to generate academic papers. Mann et al. even go so far as to claim that these LLMs can “enhance” academic prose and be useful in “idea generation”1. I argue that these bold claims cannot be correct. While we can grant that the sample texts appear coherent and may seem to contain “new ideas”, any appearance of coherence or novelty is solely “in the eye of the beholder” (Bender et al.2). Since the generated text is not produced by an agent with communicative intentions (Grice 19573) our ordinary notions of interpretation – and, derivatively, of such notions as coherence – break down. As readers, we proceed with the default assumption that a text has been produced in good faith, naturally trusting what it says to be true (absent indications to the contrary) and expecting these truths to form a coherent whole. But this default assumption is misplaced in generated texts and, if unchecked, will allow both falsehoods and inconsistencies to pass under our radar. Whatever one thinks of the use of LLMs to help create content for commercial publications, their use in generating articles for publication in scientific journals should raise alarms.
Keywords: Personalized large language model (PLLM); Machine learning (ML); Communicative intention; Encapsulation
References
- Manna SP, Earp BD, Møller N, Vynn S, Savulescu J. AUTOGEN: A Personalized Large Language Model for Academic Enhancement—Ethics and Proof of Principle. Am J Bioeth. 2023 Jul 24;1-14. doi: 10.1080/15265161.2023.2233356
- Bender EM, Gebru T, McMillan-Major A, Shmitchell S. On the dangers of stochastic parrots: can language models be too big? Proceedings of the 2021 ACM Conference on Fairness, Accountability, and Transparency. March 2021, 2021, pp. 610-623. doi.org/10.1145/3442188.34459223.
- Grice HP. Meaning. Philosophical Review 1957;66:377-388.